Forecasting is fallible, yet necessary
Economic forecasters are caught out by shocks so often that one might reasonably ask why they bother. The short answer is that they have no choice, writes ING chief economist Mark Cliffe for Project Syndicate
In a complex and uncertain world, making predictions is a fraught business, not least for economists, whose forecasts are notoriously inaccurate. Even worse, economic forecasts tend to let you down just when you need them most. The Nobel laureate economist Paul Samuelson once quipped that “the stock market has called nine of the last five recessions,” which seems forgivable when compared with economic forecasters who rarely predict any.
People simply cannot live without predictions
Given that economic forecasters are so often caught out by shocks, one might ask why they bother. The short answer is that they have no choice. Even when they are well aware of the fallibility of their analyses. People simply cannot live without predictions. Because all decisions – in business, politics, or even one’s personal life – are based on some idea of what the future holds, demand for forecasts is insatiable. People want to be able to justify decisions that they would have made anyway for other reasons. And when things go wrong, they can always blame the “experts.”
The problem of overconfidence
Forecasts – and the stories behind them – can comfort us, but they can also lead us astray by creating an illusion of control. This is why investment projects are often late or over budget. Similarly, official forecasts published by government agencies and central banks tend to be overly optimistic. This not just because they are based on assumptions of a policy’s future success, but because they are designed to change people’s expectations and behaviour.
When conjuring such self-fulfilling prophesies, forecasters often ignore the sage advice that 'it’s better to be vaguely right rather than exactly wrong'. Having constructed elaborate models to predict the future, they tend to forget that they are offering only approximations.
Of course, it doesn’t help that the most confident and provocative forecasters are the ones who make headlines. As Philip E. Tetlock of the University of Pennsylvania has shown, the most direct and simple narratives are often way off the mark. Yet, rather than “weed out bad ideas,” the media often favour them, “especially when the truth is too messy to be packaged neatly.”
We should always be wary of forecasts based on a single factor
Indeed, the devil is in the detail, so we should always be wary of forecasts based on a single factor. At the same time, we should remain open to forecasters who are honest about their degree of confidence (or lack thereof). Far from signalling weakness, such self-awareness indicates that they have based their prediction on a realistic interpretation of the data, rather than speculation and unjustified assumptions.
We all have implicit biases, and forecasters are no exception. More recent and shocking news events tend to command more of our attention than episodes in the distant past. And yet the latest news might be misleading, especially when it comes to newly published statistics that may be subject to large revisions in the future.
Moreover, forecasters, like everyone else, have a natural tendency to indulge in elegant, after-the-fact rationalisations. Yet hindsight, though a wonderful thing, poses dangers of its own. All too often, forecasters succumb to selective memory, cherishing their successes while forgetting their failures. To the extent that they fail to acknowledge and learn from their mistakes, they are prone to repeat them.
Art, Not Science
Forecasters have an incentive to make more predictions because doing so raises their chances of getting something right. But, on a more practical level, it also offers more chances to learn, assuming that forecasters are prepared to revise their views in light of new information.
Here, it is important to remember that forecasters are not playing the same game as financial market traders and investors. Because the latter are judged mainly by the profits they make, they are happy to get things right for the wrong reasons. By contrast, forecasting is not primarily concerned with accuracy per se. Nobody should expect forecasters to get it right all of the time. As long as they specify their confidence and avoid catastrophic errors, what they are really offering is a mode of thinking.
The most meaningful forecasts, then, are the ones that provide useful explanations and a cogent framework for understanding current conditions and future events. What matters is not so much the amount of information a forecaster marshals, but rather the depth of the wisdom underlying the analysis. Those who focus solely on information are liable to equate correlation with causation. Wisdom comes from a deeper understanding of why things happen.
Forecasters’ models are necessarily based on past causal relationships. People’s spending, for example, will typically reflect their incomes. But while these variables do reliably follow steady trends or cyclical patterns, history does not repeat itself or even necessarily rhyme. Just because a variable followed a certain trend in the past does not mean that it will do so in the future. “Normal” can be a moving target – models live in the past and die in the future.
Economists make a crucial distinction between risk and uncertainty
Accepting that it takes just one unprecedented shock to wreck a forecasting model, economists make a crucial distinction between risk and uncertainty. With risk, you at least know the odds, such as when playing roulette; with uncertainty, you don’t – indeed, can’t – really know anything. “Unknown unknowns,” to use former US Secretary of Defense Donald Rumsfeld’s term, could change the rules of the game at any moment. That is why forecasting will always be more an art than a science.
The further out you look, the less reliable your forecast will be, which is why economic pundits on TV prefer to appear smart by focusing only on the short term. In fact, some forecasts of monthly indicators, which are released with roughly a month’s delay, are more like “backcasts,” because anecdotal information helps to “predict” last month’s outcome.
Beyond the time dimension, some forecasting variables are more prone to extreme swings than others; for example, commodity and share prices are far more volatile than consumer prices. It is important to understand these dynamics because the price point or circumstances that inform the starting point of a forecast are often critical to the outcome.
Economies, like climactic or biological systems, can be acutely sensitive to initial conditions. The phenomenon of one thing leading to another – like the proverbial butterfly causing a hurricane by flapping its wings – can make forecasting especially difficult when such path dependencies aren’t understood at the outset.
In wrestling with the sheer complexity of the economy, the forecaster’s job is helped by the fact that some things matter more than others, and therefore deserve more attention.
Sometimes this is obvious: the prospective price of oil is a critical determinant of the profitability of an oil rig, whereas many other variables are not.
Still, many other crucial risks can evade forecasters, particularly when they are tied to deeply held assumptions that lead us to take things for granted. An obvious example of this is the 2008 global financial crisis, which owed much to the widespread complacent belief that US house prices never fall.
The Role of Stories
One way to deal with myriad possible futures is to construct scenarios. Rather than betting the farm on a single forecast, one can buy into a larger story that has been constructed around certain key drivers in terms of probability and impact. Such stories offer benchmarks for assessing what kind of a world we are in. We know that people tend to make bad decisions when under stress, so it is helpful to know if some potentially disruptive event might be in the offing. We can plan for such contingencies and aim for “grace under fire.”
That said, when thinking about alternative scenarios, it is important not to overlook the possibility of “unknown unknowns”. Ultimately, however, having too many stories will lead to confusion, so one must eventually make a choice. The goal should be to develop a “no regrets” strategy that accommodates a variety of scenarios; admittedly, this is easier said than done.
Stories are all the more important for grappling with issues that don’t lend themselves to measurement. Economists often ignore non-economic factors that are hard to include in their models, which can result in missing the elephant(s) in the room. In particular, politics and institutions matter. They can be critical in determining whether countries ultimately succeed or fail. And even in the short term, recent US experience shows that a divisive election can be far more important than a forensic analysis of the latest economic data when formulating a forecast.
Having more data isn't always better
Indeed, having more data isn’t always better. To be sure, data are necessary for testing theories in the real world; but the numbers rarely speak for themselves. Nonetheless, with economic forecasters having been thoroughly embarrassed by the financial crisis, they are in danger of being overshadowed by the new breed of “data scientists.” There is no denying that big data and machine learning are opening up new forecasting opportunities by applying massive computing power to pattern recognition and automated trial and error. But such powerful new tools can be dangerous weapons in the wrong hands.
For example, far from being a silver bullet, “predictive analytics” is really appropriate only in microeconomic forecasting. Though adding more data and variables can help identify more correlations, the proportion that are spurious and dangerously misleading rises even faster. As Nassim Nicholas Taleb puts it, big data elevates “cherry-picking to an industrial level.”
This brings us back to the distinction between wisdom and information. Failing to put data into context, check its quality, or understand the questions that one is trying to answer will result in noise, not signal. Forecasts will not be improved unless we are identifying why things are happening.
Likewise, more data in an uncertain world may create the impression that we are dealing with calculable risks when we actually are dealing with unknowns. In such situations, simple rules of thumb – or heuristics – might well outperform complex forecasting techniques.
After all, we don’t even need precise, quantitative forecasts for some purposes. Sometimes the direction is enough, such as in situations that have binary outcomes. Elections (or wars) are either won or lost. We are far more concerned with who wins than with the margin of victory.
Similarly, non-linear relationships can create major problems for forecasters, because they are difficult to model and harder still to forecast. This is particularly troubling in view of the explosive growth of many of the emerging digital technologies. Even minor initial errors in one’s forecasting model can quickly lead to huge ones.
In this environment, Taleb argues in his 2012 book "Antifragile: Things That Gain from Disorder" that we should give up on forecasting altogether, and instead focus on non-predictive forms of decision-making. That means looking for choices that would confer benefits in the event of unexpected extreme changes. But, again, this is easier said than done, especially now that many businesses appear to be paralysed by uncertainty, rather than energised by techno-phoria. That said, technological progress is not entirely unpredictable. Moore’s Law, which stipulates that computer processing power doubles every two years, has proven to be remarkably durable.
The certainty of uncertainty
Surveys of forecasts typically reveal that there are only a few mavericks willing to stick their necks out; most forecasters prefer to stay close to the consensus of their peers. They seek safety in numbers, reckoning that it is better to get it wrong in a crowd. This is just as well when it comes to the financial markets, where forecasters are typically wrong, often by large margins.
Financial markets are not like other markets, because the prices are themselves forecasts. They reflect expectations of returns and risk. According to the “efficient market hypothesis,” one cannot beat the wisdom of the crowd, because its collective intelligence already incorporates all available information. But while markets do incorporate incoming information rapidly, the hypothesis does not stand up to scrutiny. After all, the “wisdom” of the crowd is often revealed to be madness.
This makes sense when one accepts that investor beliefs about the future are loosely held, and prone to rapid swings from optimism to pessimism, resulting in booms and busts that leave consensus-minded forecasters trailing in their wake. And for their part, forecasters are prone to hedge their forecasts by saying that “times are unusually uncertain.” It is surprising how rarely this cliché gets challenged.
The world is beset by potential volatility
It is easy to forget that all eras have their own uncertainties. Today, the world is beset by potential volatility from digital technologies, political and social turmoil, unconventional monetary policies, financial fragilities, and regulatory change. But hindsight makes it easy to forget past sources of uncertainty, not least the existential threat of “mutually assured destruction” during the Cold War.
Because forecasters’ hand-wringing about “unusual uncertainty” has the practical effect of deterring risk-taking, it leads to a pessimistic outlook. Yet we should not forget that the darkest hour comes before the dawn. Positive surprises do happen.
This article by ING's Mark Cliffe was commissioned by Project Syndicate.
Tags
EconomicsDownload
Download article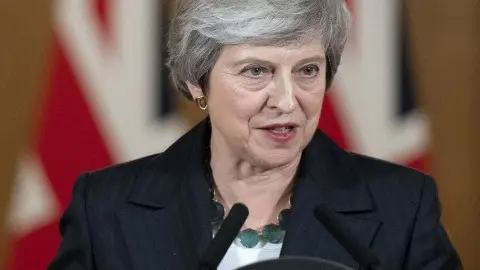
12 November 2018
In case you missed it: Peak uncertainty This bundle contains 8 Articles"THINK Outside" is a collection of specially commissioned content from third-party sources, such as economic think-tanks and academic institutions, that ING deems reliable and from non-research departments within ING. ING Bank N.V. ("ING") uses these sources to expand the range of opinions you can find on the THINK website. Some of these sources are not the property of or managed by ING, and therefore ING cannot always guarantee the correctness, completeness, actuality and quality of such sources, nor the availability at any given time of the data and information provided, and ING cannot accept any liability in this respect, insofar as this is permissible pursuant to the applicable laws and regulations.
This publication does not necessarily reflect the ING house view. This publication has been prepared solely for information purposes without regard to any particular user's investment objectives, financial situation, or means. The information in the publication is not an investment recommendation and it is not investment, legal or tax advice or an offer or solicitation to purchase or sell any financial instrument. Reasonable care has been taken to ensure that this publication is not untrue or misleading when published, but ING does not represent that it is accurate or complete. ING does not accept any liability for any direct, indirect or consequential loss arising from any use of this publication. Unless otherwise stated, any views, forecasts, or estimates are solely those of the author(s), as of the date of the publication and are subject to change without notice.
The distribution of this publication may be restricted by law or regulation in different jurisdictions and persons into whose possession this publication comes should inform themselves about, and observe, such restrictions.
Copyright and database rights protection exists in this report and it may not be reproduced, distributed or published by any person for any purpose without the prior express consent of ING. All rights are reserved.
ING Bank N.V. is authorised by the Dutch Central Bank and supervised by the European Central Bank (ECB), the Dutch Central Bank (DNB) and the Dutch Authority for the Financial Markets (AFM). ING Bank N.V. is incorporated in the Netherlands (Trade Register no. 33031431 Amsterdam).